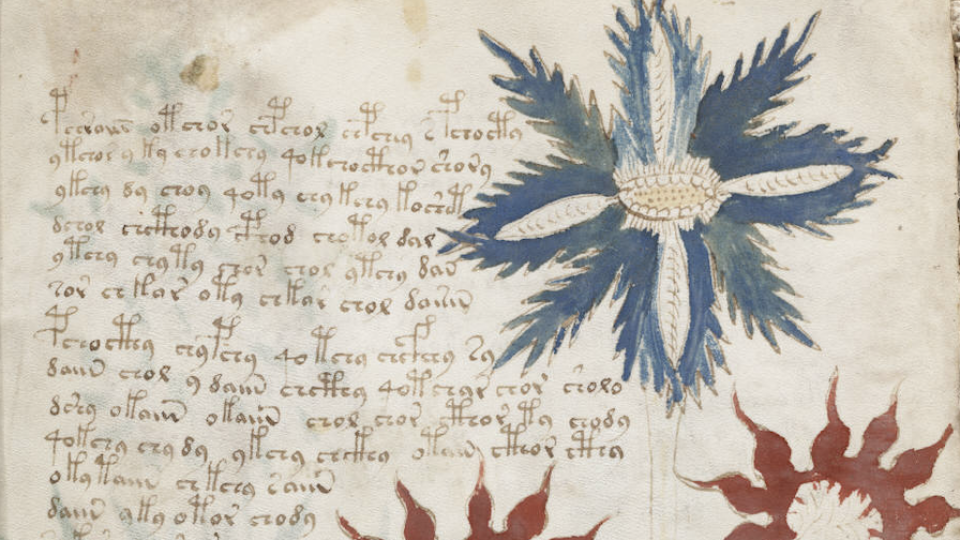
28 Maggio 11-12:30 in Aula 1.17
Adapting machine learning models for clinical language processing
Steven Bethard The University of Arizona, USA
Steven Bethard is an Associate Professor at the School of Information at the University of Arizona, where he is part of the faculty of the Computational Language Understanding Lab. His research interests include natural language processing and machine learning theory and applications, including modeling the language of time and timelines, normalizing text to medical and geospatial ontologies, and information extraction models for clinical applications. Abstract: One of the most common questions from users of clinical natural language processing software (e.g., Apache cTAKES) is “Why didn’t the software find this example of X in my data?” The problem is usually that the software’s statistical model was trained on data that does not have examples like the user’s. Fixing the problem would traditionally involve adding new examples to the training data and re-training the model. This is impractical for most users, who have limited machine-learning experience and who often cannot obtain the training data due to protected health information regulations. In this talk, I will discuss some of the challenges to building clinical language processing models that can adapt to a variety of domains, and some recent progress in machine learning techniques that can address these challenges. Examples will be drawn from my lab’s work on clinical negation detection, time expression recognition, and medical concept normalization.